

As the height increases, weight tends to increase as well. The two variables seem to have a positive relationship. For example, the observation with a height of 66 inches and a weight of 200 pounds does not seem to follow the trend of the data. We say “in general” because it is not always the case. The scatterplot shows that, in general, as height increases, weight increases. We can create our scatterplot in Minitab following these steps. We can use Minitab to create the scatterplot. These coordinates are plotted on the x-y plane. For example, student 1 has coordinate (72,200). does not mean there is no relation, only that there is no linear relation. The observations are then considered as coordinates \((x,y)\). Heres an excellent video showing a scatter diagram on steroids created by. We let \(X\) denote the height and \(Y\) denote the weight of the student. If there is no clear pattern, then it means there is no clear association or relationship between the variables that we are studying. The data can be found here university_ht_wt.txt. Suppose we took a sample from students at a large university and asked them about their height and weight.
#No association scatter plot how to#
In the next sections, we will show how to examine the data for a linear relationship (i.e., the scatterplot) and how to find a measure to describe the linear relationship (i.e., correlation).ĩ.1.1 - Scatterplots 9.1.1 - Scatterplots When we look for linear relationships between two variables, it is rarely the case where the coordinates fall exactly on a straight line there will be some error. If the slope is 0, then as one increases, the other remains constant. If the slope is negative, then there is a negative linear relationship, i.e., as one increases the other variable decreases. If the slope is positive, then there is a positive linear relationship, i.e., as one increases, the other increases. For example there is no relationship between the amount of tea drunk and level of. The slope of a line describes a lot about the linear relationship between two variables. A zero correlation exists when there is no relationship between two variables. Given two points on a line, \((x_1, y_1)\) and \((x_2, y_2)\), the slope is calculated by:
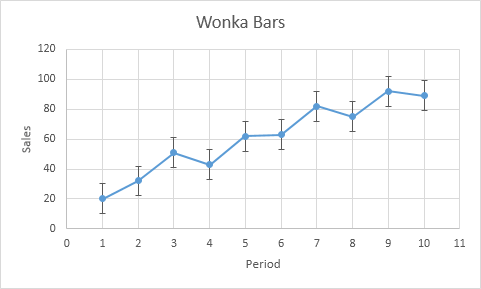
Where m is the slope and b is the y-intercept. As mentioned before, the focus of this Lesson is linear relationships.įor a brief review of linear functions, recall that the equation of a line has the following form: For example, the relationship shown in Plot 1 is both monotonic and linear.To define a useful model, we must investigate the relationship between the response and the predictor variables. The Pearson correlation coefficient for these data is 0.843, but the Spearman correlation is higher, 0.948.

This relationship is monotonic, but not linear. Plot 5 shows both variables increasing concurrently, but not at the same rate. In a linear relationship, the variables move in the same direction at a constant rate. In a monotonic relationship, the variables tend to move in the same relative direction, but not necessarily at a constant rate. You want a moderately strong association. Their plots to three and four are moving in a linear pattern for party. It can be loose, or it can be really tight. Data points move in a group stretched out in a straight form. This relationship illustrates why it is important to plot the data in order to explore any relationships that might exist. A scatter plot with the linear association will have it. Sometimes a scatter plot shows an association that is not linear: We call such an association a non-linear association. However, because the relationship is not linear, the Pearson correlation coefficient is only +0.244. Plot 4 shows a strong relationship between two variables. This curved trend might be better modeled by a nonlinear function, such as a quadratic or cubic function, or be transformed to make it linear. If a relationship between two variables is not linear, the rate of increase or decrease can change as one variable changes, causing a "curved pattern" in the data. The Pearson correlation coefficient for this relationship is −0.253. They do not fall close to the line indicating a very weak relationship if one exists. Sometimes we see linear associations (positive or negative), sometimes we see non-linear associations (the data seems to follow a curve), and other times we don't see any association at all. The data points in Plot 3 appear to be randomly distributed. Practice identifying the types of associations shown in scatter plots.
